AI for every business
27 March 2023
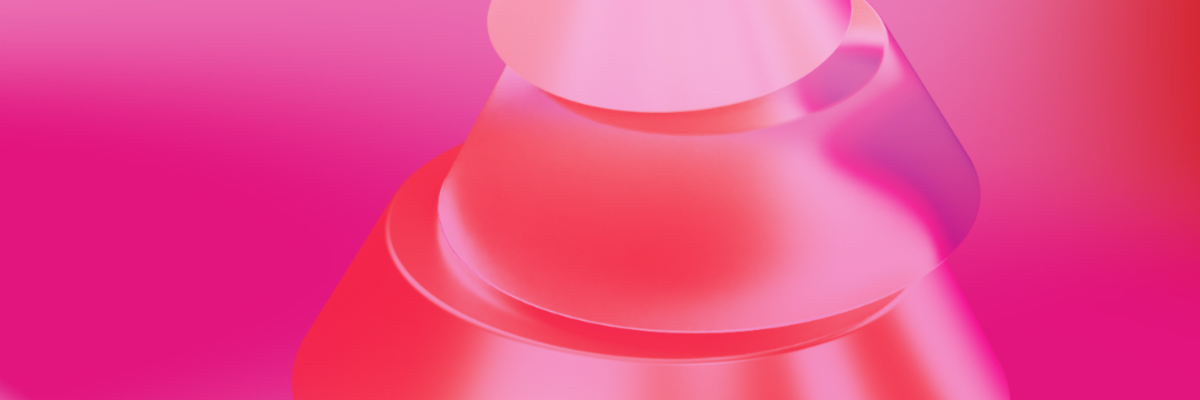
How can we successfully generate business value from Artificial Intelligence? As we wrote before, that’s the main question any business should ask itself when looking at AI.
Let’s get you started with AI and machine learning
And while we believe that every business can benefit from AI technologies, we admit it is not easy to get started. So, the least we can do is provide practical examples of how AI technology and machine learning algorithms can be applied to various business domains.
Before we dive into those examples, let's clarify what machine learning is and how it relates to AI. Machine learning is a subset of AI that trains computer algorithms to perform tasks based on data without being explicitly programmed to perform that task. And when we refer to data in this context, we're talking about many things: numbers, words, text files, sound clips, images ... you name it. If you can store it digitally, machine learning algorithms can handle it.
The key takeaway is that machine learning algorithms are designed to learn from examples and patterns in available data. They then use that learning to make predictions or decisions based on new, previously inaccessible data.
Machine learning flavors
Machine learning comes in three flavors: supervised, unsupervised, and reinforcement learning.
Supervised learning algorithms are trained on labeled data, meaning data where the correct output or "answer" is already known. Those algorithms learn to map inputs to outcomes based on the labeled example data. Classification, regression, and time series analyses are a few examples of widely used supervised learning algorithms.
In unsupervised learning, the algorithms are trained on data with no labels. Those algorithms learn to identify patterns or structures in data without explicit guidance because no known output or "answer" is available with the data. Examples include data clustering, outlier detection, and some text and image models.
Reinforcement learning algorithms, finally, learn to make decisions by trial and error to achieve a well-defined objective. Depending on whether those trials take them closer or further away from the specified objective, the algorithms get rewarded or penalized. Reinforcement learning algorithms focus on maximizing their rewards over time by learning from the consequences of their actions. Ever heard of AlphaGo? It’s the first computer program to defeat a professional human Go player (the 3,000-year-old Chinese board game), and it’s powered by reinforcement learning.
Practical AI use case examples
Time to dive into some practical examples. We’ll do that using the AI use case matrix, which you can download when clicking following link, which outlines 64 unique AI & machine learning applications, 8 of which we’ll discuss in more detail. We built this matrix by identifying 8 different business domains and 8 different tasks that can be powered by machine learning algorithms.
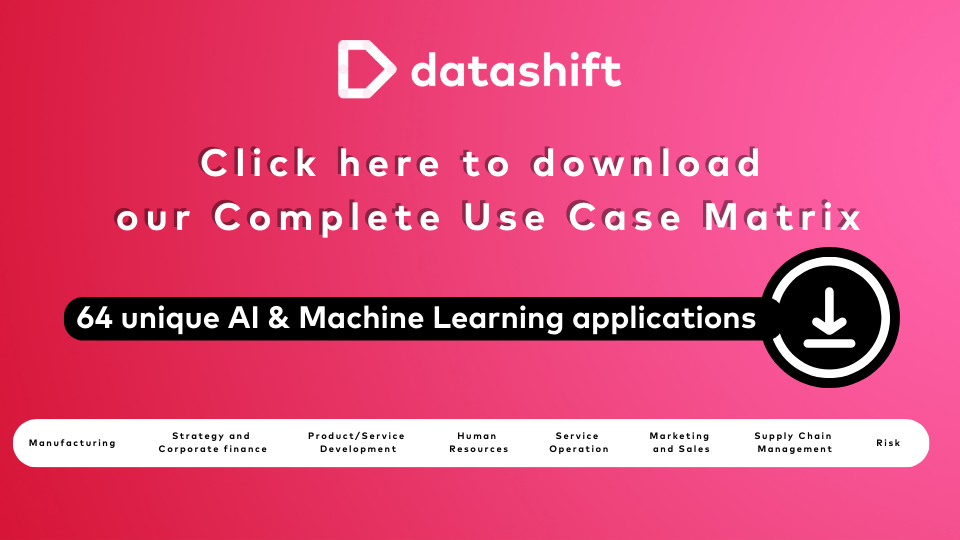
This matrix is not an exhaustive list of AI use cases, nor do the examples represent the only way to solve the related business challenges. However, those examples should give you a pretty good idea of what you can achieve with AI technology. Hopefully, they can serve as a springboard for applying machine learning to your business.
Predictive maintenance | Classification algorithms in manufacturing
As classification algorithms learn to group objects according to predefined categories based on several variables, you can use them to assess when machines need maintenance. For example, the likelihood of a part needing replacement or maintenance work can be predicted based on input variables such as the number of products made, air pressure, or oil level. Such predictive maintenance practices make it possible to achieve operational optimization in manufacturing. The result: lower downtime and fewer costs.
Business valuation in M&A | Regression models in strategy
Another use case relates to business valuation in the context of mergers & acquisitions (M&A). Suppose you use regression models in your business development strategy and corporate finance practices, for example. In that case, you can adopt such models to estimate the value of a business based on historical M&A deals. This helps avoid paying too much or selling too cheap.
Obviously, it’s not just big corporations that can develop such models in-house to improve their M&A capabilities. Consulting companies can also leverage this technology to strengthen their M&A services, while it is even possible to build a startup focused on selling business valuation predictions using dedicated regression algorithms.
Product sentiment assessment | Time series analysis in product development
As soon as you measure data points on evenly spaced time intervals over a more extended period, you are building a time series. You can then use supervised machine learning algorithms to understand the underlying trends in your data over time and even forecast future values.
For example, if you are a product owner, you can use a time series analysis to measure and predict customers' sentiment towards your product. In addition, you can research how customer sentiment changes over time and investigate the impact of external triggers such as marketing campaigns or public events. That knowledge is invaluable in making better decisions for your product development.
Identification of corporate skill gaps | Clustering in HR
Even HR management can benefit immensely from the use of AI. That may seem surprising at first glance, but don't forget there is both a soft and a hard side to HR. For example, unsupervised machine learning algorithms can help you cluster employees based on their skills, creating groups of similarly skilled employees considering various parameters. You can then use those insights to identify any existing skill gaps, research how the available skill sets could be optimized (potentially even triggering innovation initiatives), or build a skill-based compensation plan for your employees – to give a few examples.
Improved customer interaction | Outlier detection in service operations
Unsupervised machine learning algorithms are not just good at clustering highly similar data points. They can also be valuable in detecting extreme data points or outliers in your business processes. Think, for example, about how you could strengthen your customer support services using outlier detection. Once you have identified customers who’ve had extremely good or bad experiences with your services, you can use those cases to better understand how your customers interact with your support services and improve your service operations from that point forward.
Personalized messages for customers | Text models in marketing
If you are a marketeer, you know how hard it is to deliver customized messages to your customers. Setting up the proper personalization rules for various customer interaction scenarios (and understanding what works and what doesn't) is daunting. That's where machine learning can come to your rescue, and text models in particular.
From your customers' interests, text models can deliver personalized marketing messages for individual customers – potentially eliminating the need to set up loads of personalization rules. As more and more customers expect a personalized experience over time, machine learning can undoubtedly become an exciting tool to meet that expectation.
Inventory checks | Image models in supply chain management
Machine learning models can not only work with text, but they can also extract information from images or even create images. One way you could benefit from this is to use image models in supply chain management.
If you can access images or video footage of your in-stock products, you could use image models to count the number of items on a pallet. That would assure highly accurate stock inventory information without much manual effort on your side, lowering inventory management costs.
Optimized hedging strategies | Reinforcement learning in finance
Unlike the supervised and unsupervised machine learning algorithms used in all previous use cases, reinforcement learning algorithms do not require data sets to be available for training. Instead, reinforcement learning algorithms learn how to act in a specific environment by extracting rewards or penalties from that environment depending on the outcome of their actions.
In finance, you could use reinforcement learning to train a hedging module to develop an optimal hedging strategy based on information about the market and the specific risks a company is facing, safeguarding its production and value-creation processes.
So how could AI change your business?
As said before, those 8 AI & machine learning applications (along with the ones provided in the use case matrix) do not provide an exhaustive overview of AI use cases. In fact, it's not that difficult to come up with many other valuable use cases. The most challenging part is identifying which projects to pursue, given the limited resources you have access to.
So have you thought already about how AI could change your business? Which machine learning projects would create the most significant impact from a financial and strategic point of view? And how could you start creating value as soon as possible? These are not easy questions to answer. But be aware that we are here to help you with that. We’re just an email away.