How not to fail your AI projects
29 June 2023
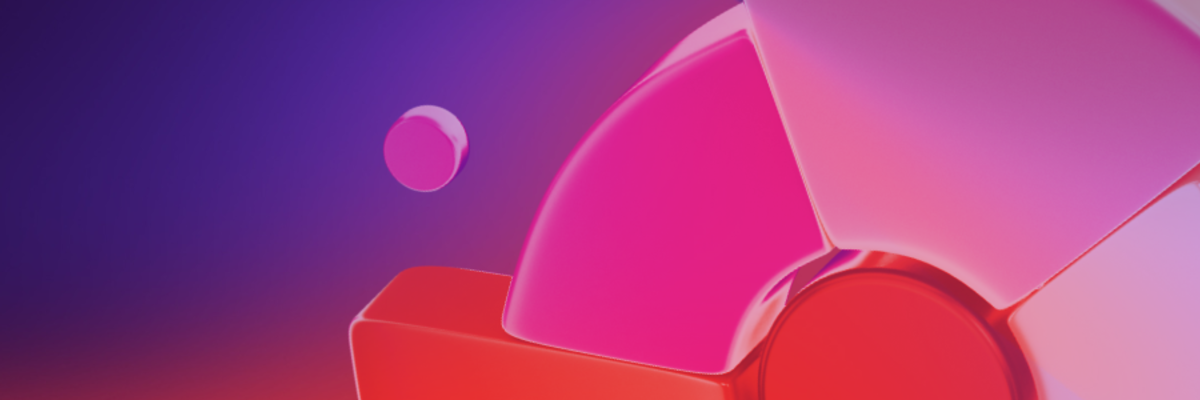
In today's world, stories about organizations achieving remarkable success with AI solutions dominate our news feeds, social media platforms, and marketing campaigns. These seemingly magical AI systems promise to revolutionize our lives by solving complex problems. However, the reality is far more nuanced. Amid the success stories, numerous companies find themselves trapped in nightmarish situations, struggling to make AI work for them.
What separates the winners from the losers? The answer lies in one key factor: data quality.
The Significance of Data Quality
It is no secret that data serves as the foundation for leveraging algorithms and deploying effective AI models. While this fact is well-known, many organizations unfortunately overlook the critical importance of data quality and accessibility. Experts in data engineering, machine learning, and data science unanimously agree that data quality is an essential element for success. Yet, when asked if they would willingly tackle data quality, most professionals would rather avoid it altogether.
The reasons for this avoidance are simple: data quality is often seen as an unappealing task, demanding significant effort, and yielding slow results. But why is that?
The Organizational Effort
To achieve high data quality, an organization-wide effort is necessary. Data comes from various sources such as customers, call centers, contracts, machines, and sales departments. Almost every job within an organization contributes to data creation. To attain superior data quality, it is crucial to involve everyone in this effort. However, this is just the beginning. Data should not only be accurate and complete when received, but it must also be continually maintained for reliability, validity, and relevance.
Unfortunately, data quality is often perceived as a cost center, lacking in value creation. The prevailing attitude is to leave data-related tasks to the individuals working on specific projects. Yet, as an increasing number of organizations have discovered, neglecting data quality leads to significant problems. The cost of ignoring data quality far surpasses the investment required to implement proper measures.
In essence, the problem lies not with AI itself, but with the available data.
The Need for Data Governance Framework
Addressing questions regarding data quality and its impact demands a comprehensive data governance framework that outlines rules, roles, and responsibilities. If you are still reading, you likely fall into one of two categories: either you work in data governance and data quality or you understand the consequences of neglecting these vital elements.
Failures and Successes
While successful AI solutions make headlines daily, the failures and struggles organizations face in implementing AI often go unreported. For every triumph, there are numerous failures. Though not all failures can be attributed to data quality issues, personal experience indicates that data quality often serves as an early indicator of project outcomes. The availability of readily usable datasets with clear documentation and high quality is a key differentiator.
Investing in proper data quality initiatives lays the foundation for successful ventures in the world of AI.
Conclusion
If you find yourself struggling to make your data projects, AI-related or otherwise, successful, it is essential to reflect on the time and effort you have dedicated to data quality. Neglecting this crucial aspect can significantly hinder progress. Conversely, for organizations experiencing AI success, it is crucial not to overlook the contributions of those individuals who have worked diligently to improve data quality and governance. Their efforts have paved the way for the success you are currently enjoying.
Now if you want to know the status of your organization's Data Quality (and therefor chance on AI success), we have something for you: Datashift's Data Quality Assessment. Test if your organization is ready for AI!