The future of Data Quality & Collibra DQ
10 March 2022
Over the past couple of years, our experienced consultant Evert has witnessed the growth of our Collibra Data Governance projects up close. So how does Evert, who knows Collibra inside and out, see the future of Collibra DQ, a Collibra platform solution that goes hand in hand with the Collibra platform, and Data Quality in general? And what are the most significant opportunities for Collibra DQ, in his opinion? Let's see what Evert has to say about all this.
Datashift: Evert, how did you get involved with Collibra in the first place?
Evert: In hindsight, that happened somewhat by chance. In my previous job as a salesman, I’d seen many customers struggle with managing the data they were responsible for. That got me thinking about exploring new professional opportunities in data consultancy. Around the same time, there was quite some talk in the newspapers about Collibra being the first Belgian unicorn, which further sparked my interest in data and data governance consultancy.
When I got in touch with Datashift during my job search, it quickly became apparent that we were a good match – even more as Datashift had recently embarked on a series of Data Governance projects using Collibra.
That’s how I rolled into Collibra, and – honestly speaking – my timing couldn’t have been better. Together with the team, I’ve thrown myself into Collibra - figuring out new possibilities and building up expertise. Since then, I’ve worked on Data Governance projects for many national and international clients – including financial institutions and manufacturing companies from various industries.
Datashift: What exactly have you been working on over the past years? And how did Data Quality come into the picture?
Evert: My main focus has been on the Collibra back-end and workflow development in particular. I've always found that very interesting because it enables me to combine my passion for building tangible solutions with a natural curiosity to understand how business processes work. Along the way, my Datashift teammates and I dived into the Collibra API and the wonderful world of Python because a growing number of clients are asking to integrate Collibra with other business tools.
Another exciting development that has impacted our Collibra projects is the acquisition of OwlDQ by Collibra in early 2021 and the subsequent addition of this Data Quality solution to the Collibra portfolio. In short, Collibra DQ (as it is called now) uses Machine Learning to monitor data quality on large data sets by learning through observation rather than human input. That makes it possible to enrich the standard technical metadata stored in Collibra with additional information on data quality coming from Collibra DQ.
As the Premier Collibra partner in EMEA, Datashift has been part of the DQ training organized by Collibra following their OwlDQ acquisition. Since then, we have started assisting clients in deploying Collibra DQ.
Datashift: So you do see a substantial market demand for solutions such as Collibra DQ?
Absolutely. The projects we’ve performed over the past year are unmistakable signs that Data Quality is a hot topic right now. Many manufacturing companies, financial institutions, energy providers,… are well aware of the problems with the quality of their data. As a result, they understand there is a tremendous amount for them to gain in managing and improving that quality.
On the other hand, it is essential to understand where Data Quality technology stands today and how it can deliver its promise. Even technologies that are well established by now, such as Big Data and AI, have initially gone through phases of inflated expectations and subsequent disillusionment. Since that's no different for Data Quality technology, companies such as Collibra are undertaking significant and structural developments in this area. Go-getters like Collibra are crucial in maturing new technologies and enabling their mainstream adoption.
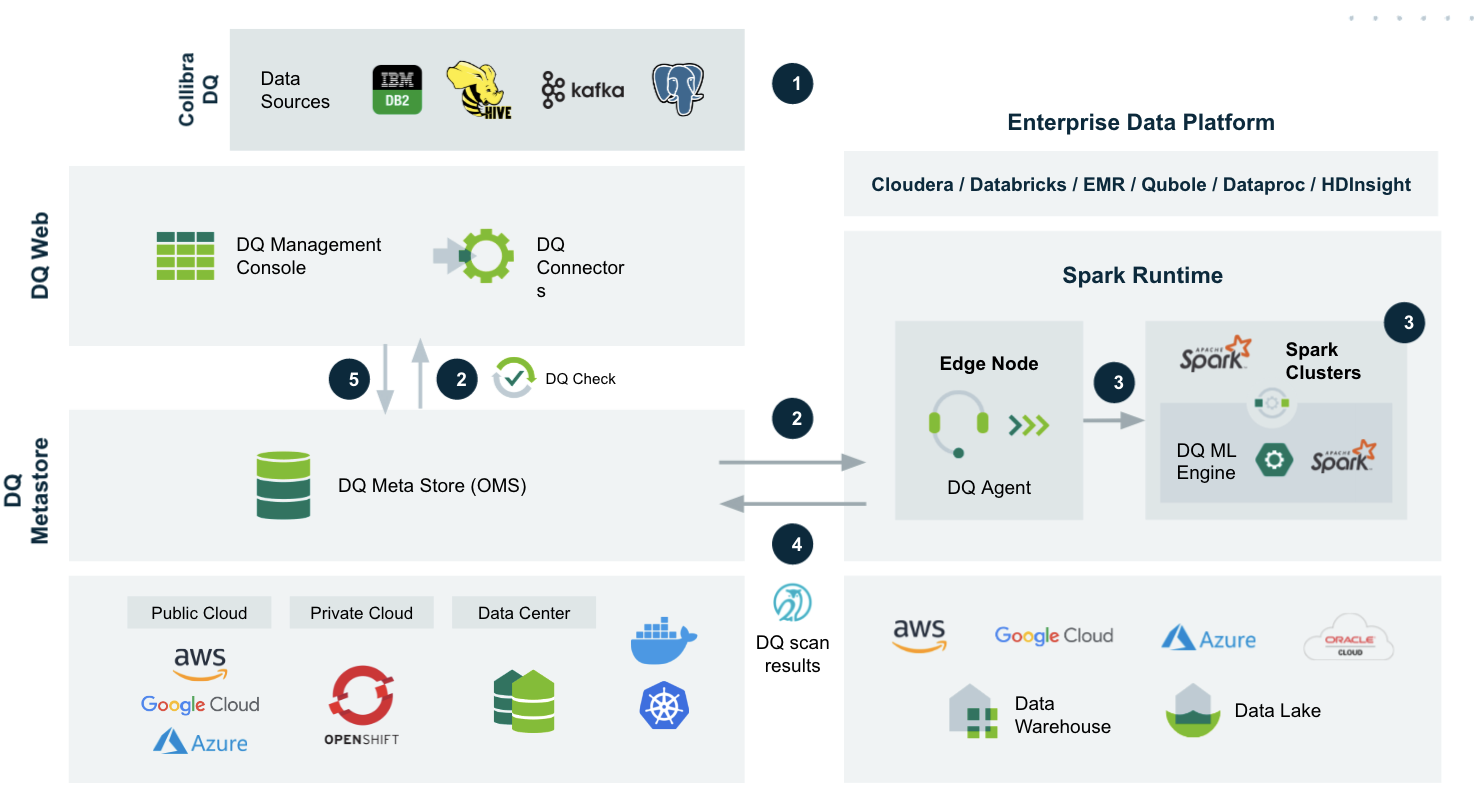
Datashift: You already highlighted what Collibra DQ does. Can you explain that in more detail and provide some concrete examples?
Evert: Sure. The Internet of Things, or IoT, provides us with many excellent examples illustrating the importance of Data Quality. IoT devices collect numerous amounts of operational data, whether temperature measurements on wind farm turbines or measurements on an airport runway, to give a few examples. But what happens if IoT sensors fail for one reason or another, or transmit erroneous measurement data?
While business users may define rules such as “temperature measurements must lie between -20°C and +50°C”, documenting all those rules manually in Collibra takes far too much time to detect anomalies in the massive amounts of IoT data. If such anomalies go unnoticed for a few days, it doesn’t matter so much. But if they persist over a more extended period, or in bulk on multiple sensors, the decision models built on those data will sooner or later show significant aberrations.
That's precisely where Collibra DQ comes in. Collibra DQ uses machine learning to infer the data quality rules, that would be too much effort for business users to document, from the data itself. Afterwards it flags anomalies/issues it sees.
Datashift: Based on your experience, where do you see the most significant opportunities for Collibra DQ in the near future?
Evert: I see opportunities at different levels, actually. To start with, it is still quite a challenge to build Machine Learning models that automatically infer the appropriate Data Quality rules from the data. However, that challenge presents some excellent opportunities for developments focused on extending Machine Learning models.
Another significant opportunity, in my opinion, relates to making it easy for business users to engage with Data Quality rules. Just like they can now enter semantical metadata in Collibra, they should be able to implement the proper Data Quality rules by themselves. Again, let's go back to the example of the airport. If you have a higher number of planes coming in during a given period, you expect the number of passengers to go up as well. This indirect link between the number of incoming aircraft and passengers is part of your data's DNA. So, in the end, you would like your business users to define Data Quality rules such as “I expect that, allowing for a given tolerance, the number of incoming passengers goes up as the number of incoming planes increases."
Those rules are very interesting because they can timely, or even proactively, alert any issues that may otherwise remain unnoticed for a while. For example, if an ETL is failing somewhere, there is simply no way to know it in time without any such rules in place. And, let’s be honest, who is better placed than the business user to understand which Data Quality rules are most relevant?
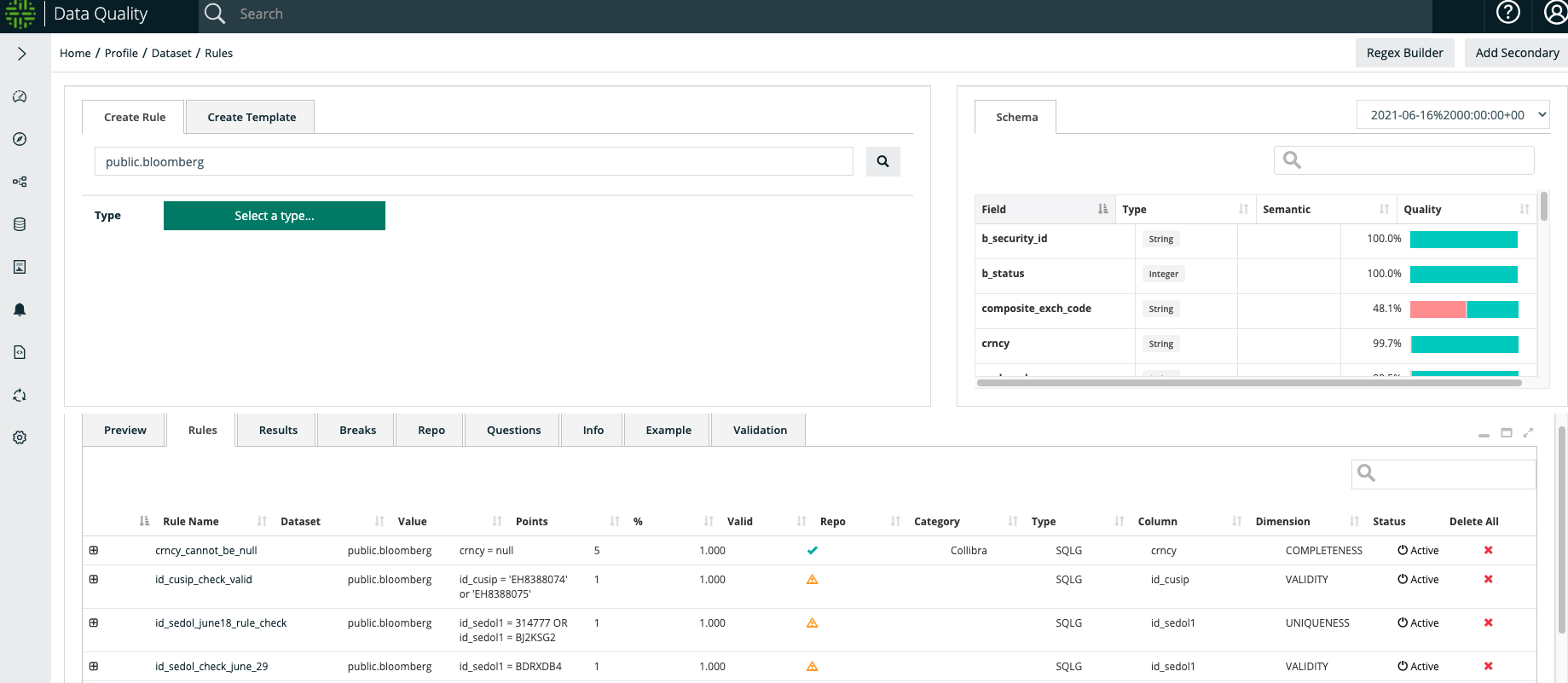
Datashift: Talking about business users: what about the integration between Collibra and Collibra DQ?
Evert: While Collibra & Collibra DQ are still two separate tools today, a lot of development work is ongoing to integrate both tools. That integration will enable the exchange of Collibra metadata with Collibra DQ and – reversely – automatically feed results from Collibra DQ back into Collibra. With full integration between Collibra and Collibra DQ in place, the intend is that business users will effectively be able to set up their Data Quality rules through the user-friendly Collibra front-end.
Somehow, history keeps repeating itself. Just as I threw myself into Collibra a couple of years ago, I’ve now been doing the same thing with Collibra DQ (together with my Datashift teammates, of course). Knowing what a company such as Collibra is capable of, I'm looking forward to seeing Collibra DQ evolve and grow over the next couple of years.